Data Helps Explain the Mysteries of Crystal Formation in the Atmosphere
New research significantly increases the accuracy of weather forecasting
Add bookmark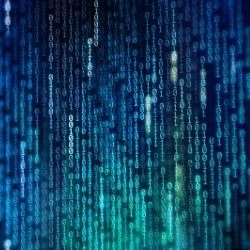
Researchers have used deep learning to understand how ice crystals form in the atmosphere. The new paper, published this week in PNAS, will potentially fundamentally alter how meteorologists predict the weather and climate forecasting.
The latest model works via deep learning to better understand and predict how atoms and molecules behave. The model was trained on small-scale simulations of water molecules in order to understand how the electrons within their atoms interact. These models then replicated the findings at a larger scale, and consequently allowed the team to accurately predict physical and chemical behaviour.
The lead author on the study, Pablo Piaggi – a research fellow at Princeton University – said: “The properties of matter emerge from how electrons behave. Simulating explicitly what happens at that level is a way to capture much more rich physical phenomena.”
According to the paper, it is the first time this method has been used to model something as complex as the formation of ice crystals, also known as ice nucleation. This is one of the first steps in the formation of clouds, which is where all precipitation comes from.
Currently, ice nucleation is currently predicted on the basis of laboratory experiments. Researchers collect data on ice formation under different laboratory conditions and then feed that data into weather prediction models using similar real-world conditions.
While this method works to a degree, the reality is that much weather forecasting is cast adrift by the multitude of external factors influencing it. By predicting ice nucleation from the way electrons interact, weather forecasters can be more precise.
Historically, this has been computationally expensive. Historically, this has been computationally expensive. And research has often taken years to actually materialize into anything useful. In fact, even with the longer timescale, the result has never before been able to analyze developments on a molecular timescale.
However, by using deep learning, researchers were able to run the calculations in just 10 days, at a time duration which was 1,000 times longer—still a fraction of a second, but just enough to see nucleation.
Future research will be to take this even further, by incorporating factors such as levels of smoke in the air to lead to even greater accuracy. As Piaggi says: “That has opened essentially a new field. It’s already having and will have an even greater role in simulations in chemistry and in our simulations of materials.”