AI Democratization Part Two: Data Governance as the Engine Powering AI Success
Future winners of the race towards AI are investing in data governance and democratization now
Add bookmark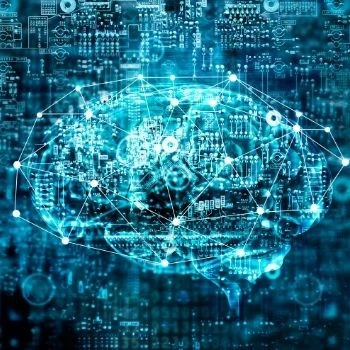
READ PART ONE >> Are Citizen Developers the Key to Applied AI Success?
Data governance, or lack thereof, can make or break an artificial intelligence (AI) model. Afterall, as the frequently quoted business adage goes, “bad data in means bad data out.”
Data governance (DG) is defined as "a system of decision rights and accountabilities for information-related processes, executed according to agreed-upon models which describe who can take what actions with what information, and when, under what circumstances, using what methods." Traditionally, DG has focused on operational aspects of the data lifecycle such as master data management, data quality, and data retention. In recent years, the scoop of DG has also expanded to include data privacy and security as global regulations in this area have evolved.
However, now leading-edge organizations are looking towards DG as a powerful tool for optimizing the data to machine learning (ML) pipeline while also promoting ethical AI. As Technology Strategist Brian Ka Chan puts it, “Starting an AI project without checking your data first is like building an F-1 race car without understanding what quality, type, specification, or sustainability of fuel you want to use.”
WATCH NEXT: Democratizing AI with External Data
In a recent whitepaper by Dataiku and Capgemini, “From Data Governance to AI Governance: How to successfully make the shift?,” they argue that “responsible AI” governance frameworks should aim to answer the following questions:
- What data is being chosen to train models, and does this data have pre-existing bias in and of itself?
- What are the protected characteristics that should be omitted from the model training process (such as ethnicity, gender, age, religion, etc.)?
- How do we account for and mitigate model bias and unfairness against certain groups?• How do we respect the data privacy of our customers, employees, users, and citizens?
- How long can we legitimately retain data beyond its original intended use?
- Are the means by which we collect and store data in line not only with regulatory standards, but with our own company’s standards?
The goals of AI governance are to ensure:
- Ethical AI - models operate without bias toward a specific group
- Efficient AI - models deliver provable, real-world business value
- Explainable AI - organizations have to be able to explain how the model made its decision, and why. This ensures AI models can meet a growing list of regulations such as GDPR.
Building a Governance-Centric Data Culture
If data is the fuel that drives AI and ML forward, citizen developers and analysts are the ones in the driver's seat determining whether the project steers ahead or crashes into the crowd. In this scenario, one could think of data or AI governance as a guardrails or a roadmap.
In addition to a set of rules or framework, it’s often helpful to think of data governance as an ongoing conversation. While ensuring that low-code enabled citizen developers and the larger workforce embrace DG is incredibly important, so is working alongside them to evolve your approach over time.
One company that has elevated data governance into a key enabler of strategic value is DNB Bank. Seeing as though people rely on financial services firms such as DNB to manage their livelihoods, instilling customer trust is a topmost priority as is making evidence-driven decisions that minimize risk.
In an interview with FinTech magazine, Aidan Millar, Chief Data and Analytics Officer at DNB, explained, ““The ethical use of data will be a strategic differentiator for banks of the future. Companies that haven’t used their data in an ethical way will lose trust, and one bad customer experience can mean that trust is lost forever.”
With that in mind, DNB took a federated approach by distributing data stewardship responsibilities across the organization and strived to establish a strong ownership culture among people at all levels to ensure full engagement. It’s also made governance a core component of its widely circulated, “ethical by design” AI strategy.
Become a Member of the AI, Data & Analytics Network so you don’t miss any of these updates.