How Spotify Uses Data to Keep You Listening
A quick look at Spotify's approach to data monetization, visualization and personalization
Add bookmark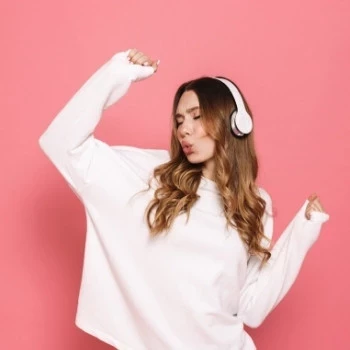
With over 320 million monthly users and home to 60 million tracks, four billion playlists and 1.9 million podcasts, Spotify is one of the most popular music (and, increasingly, talk content) streaming platforms in existence.
Similar to its big tech rivals and partners, much of Spotify’s success has been fueled by data and analytics. By collecting and analyzing massive amounts of listener data (music taste, tracks, artists, albums) Spotify can identify emerging user trends in real-time and rapidly develop new features or services to capitalize on them.
Personalization Pioneers
While Spotify does generate some revenue from paid advertisers, the bulk of its revenue comes from paid premium subscriptions. As a result, Spotify is highly incentivized to leverage customer data in a way that enhances and further monetizes the user experience vs. enables advertisers to better target potential customers.
“It is our smartest way to learn more about our audiences and our most effective way to better serve them. That data, usage, and behavior gives us all of the richness that helps us literally build product and follow the behavior to serve in a way that offers value and works in service of people, their time, their behaviors, and their moods, instead of just trying to build an ad-revenue-oriented business. We are focused on the user experience, and the free business allows us to do that at scale,” Spotify’s former global head of verticals Khartoon Weiss explained in a 2019 interview.
One of Spotify’s major competitive advantages is it’s formidable recommendation engine. Using machine learning (ML) algorithms, natural language processing (NLP) and convolutional neural networks (CNN), Spotify is able to transform historical listening data into personalized playlists and music recommendations.
To break down Spotify analytics further, Spotify uses ML to analyze user behavior and group people together based on musical preferences. Using this information, it can recommend listeners songs based on what “similar” users are also listening to.
On top of that, they use NLP to scan thousands of articles, blog posts and message boards to analyze language used to describe certain artists and songs. Using these insights, they can then group together music based on the words and phrases used to describe it (i.e. smooth jazz, melodic rap, classic rock, etc.) which helps Spotify more easily identify similar artists and build personalized playlist around them.
Finally, using CNN, Spotify analyzes raw audio data such as the song’s BPM, musical key, loudness, etc. Using this information, it can then classify songs based on music type and further optimize its recommendation engine.
*Image sourced from “How Spotify know a lot about you using machine learning and AI.”
According to a 2019 Data Science Central article, Spotify’s “home screen” uses a ML algorithm known as BaRT to provide listeners with music recommendations in real time. BaRT, which stands for Bayesian Additive Regression Trees, is unique in that:
- It is optimized for >30 seconds streams. Meaning that any song you listen to for less than 30 seconds is ignored
- They retrain the model once a day based on interaction data collected
- The system was built to de-bias for positional bias. Meaning that clicks on the bottom of the page are weighted higher than those at the top
As for next steps, in January of 2021, Spotify was granted a new patent for an AI application that uses voice data to determine users’ “emotional state, gender, age, or accent” and then leverage these insights to enhance music recommendations.
Creating Memorable Listener Experiences
In addition to building a powerful audio content recommendation engine, Spotify has developed a number of other creative ways to monetize customer data and create memorable user experiences.
For example, Spotify Wrapped uses data visualization to transform raw listener data into a compelling, personalized story that recounted each user’s listening habits and streaming history over the past year. Not only does Spotify Wrapped showcase which songs the user listened to the most, but also gave listeners “badges” that signified certain accomplishments (i.e. if a number of their playlists gained significant new followers, they’re given a Tastemaker or, if they listened to a hit song before anyone else, they were given a Pioneer badge.)
READ NEXT: Tesla: Automaker or Data Company?
“Rather than simply reminding people of great music through a 30-second spot, [we wanted to use] the data to remind people about the specific role music plays in their lives,” Spotify CMO Seth Farbman commented all the way back in a 2015 interview.
This data-driven innovation is also enhancing the artists’ user experience as well. For example, "Plagiarism Risk Detector And Interface" uses AI to detect plagiarism in a musician's lead sheets. Spotify also using data wrapping techniques to, essentially, create an analytics platform where artists can access an “easy-to-understand breakdown of playlist categories (algorithmic, editorial, and listener generated) and absolute counts for listeners and streams of an artist’s tracks on those playlists,” according to the bloggers at “How Music Charts.”