The 5 Data and Analytics Trends that will Reign Supreme in 2022
Add bookmark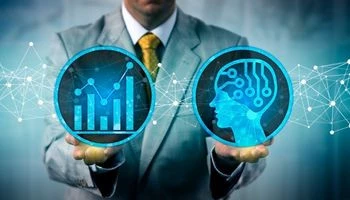
2022 is poised to be the biggest year for data and analytics yet. Here’s a look at 5 of the biggest trends shaping the industry. For those looking for more in-depth analysis, download our 23-page 2022 Outlook report HERE.
Edge + 5G = The Next Generation of Real-Time Data and Analytics
While 5G increases speeds by up to ten times that of 4G, mobile edge computing reduces latency by bringing compute capabilities into the network, closer to the end user. The highly potent combination of edge computing and 5G will enable organization process and analyze incredibly high volumes of data at breathtaking speeds.
The marriage of mobile edge computing and 5G will likely accelerate innovation in numerous cutting edge fields such as IoT analytics, augmented/virtual reality, intelligent automation and high-fidelity digital media.
Small Data and Causal AI
Organizations are rapidly discovering that when it comes to data and analytics, bigger isn’t always better. Not only are smaller data sets easier, not to mention cheaper, to manage, secure and process, they can have an outsized impact on the business. For example, small data can produce the types of granular insights that drive hyper-personalization.
While most AI platforms were built to run on large, fast moving data sets, casual AI is emerging as a potential tool for analyzing and capitalizing on small data sets. While most AI algorithms aim to predict outcomes, causal AI algorithms seek to uncover cause and effect.
Though causal models require less training data and are a key step towards achieving general AI, few have successfully deployed causal AI in real-world environments. However, given the pace at which AI innovation is accelerating, we expect to see more and more successful causal AI projects over the next year.
From Data Fabric to Data Mesh
The days of monolithic, centralized data platforms are over. Organizations, especially those with a need to access enterprise data from across multiple locations spanning the globe, are embracing data fabric solutions. Essentially a distributed data platform, data fabrics combine various types of data storage, access, preparation, analytics, and security tools into a single, consistent data management framework.
Unlike traditional architectures, data fabrics not only support large volumes of data from multiple locations, it increases the speed at which it can be accessed. However, data access remains centralized.
While data mesh seeks to solve the same problems (managing high volumes of data across multiple locations), it does so in a different way. Envisioned by Zhamak Dehghani in 2019, data mesh is “an intentionally designed distributed data architecture, under centralized governance and standardization for interoperability, enabled by a shared and harmonized self-serve data infrastructure.”
As more and more organizations transition to a hybrid cloud model, data mesh will become more widely adopted as each node has local storage as well as computational power and no single point of control (SPOC) is necessary for operation.
For many mature organizations, data mesh will be approached as a complementary technology to data fabrics. However, as data fabric solutions still require extensive ML integrations, some may choose to forgo it and embrace data mesh on its own.
Composable data and analytics
Gartner defines business composability as “the mindset, technologies, and set of operating capabilities that enable organisations to innovate and adapt quickly to changing business needs.”
As applied to data and analytics, “composable” represents a more modular, and thus agile, approach to data science. In other words, the cultivation of a data system that contains sub-components that can be quickly and easily selected/assembled in a multitude of ways to satisfy specific user requirements.
Low code analytics tools are expected to be significant enablers of composable data science as it allows users to quickly build and operationalize analytics products.
Unstructured Data monetization
As unstructured data processing technologies such as computer vision, IoT and natural language processing (NLP) evolve, the opportunities to capitalize off these innovations will erupt. In fact, according to our own research, 48% of data and analytics leaders expect to increase their investments in data monetization.
Many of the data monetization efforts will involve the creation of data-enabled products. For example:
- 7-Eleven recently opened its first computer vision-powered cashless convenience store customers to put goods in their basket and head straight out the door
- Netflix just announced it will add a voice assistant provided by SoundHound to its new Da Vinci Reference Design Kit (RDK) product. Using this new tool, viewers will soon be able to request content using adjectives like funny or heartwarming as well as by specifying a performer then following up for specific release dates or lengths.
- Connected-coaching company Asensei recently released (App)erture, a computer-vision enabled product that takes video images of athletes performing and converts human motion into skeleton data that will track form, count reps and provide coaching cues
A major offshoot of UDP that’s poised to take off is generative artificial intelligence, AI technology that can use existing content like text, audio files, or images to create new plausible content. At first glance this may not seem so exciting (do we really need more bad YouTube videos?). However, it is actually quite revolutionary.
In addition to enhancing image processing and film restoration projects, it’s also being used to build better prosthetics and protect the identity of whistleblowers.
Honorable mentions: Chief Data Ethics Officers, Data Science for Social Impact, Low Code AI, Environmental AI, the Rise of Data Marketplaces and Exchanges,