Building an Infrastructure for a Multimodal World
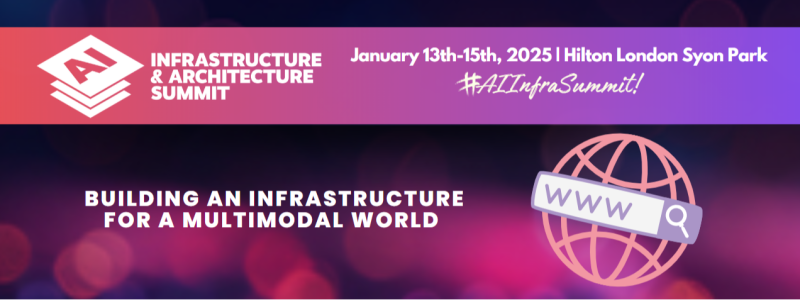
If you thought that the world of artificial intelligence (AI) was beginning to settle down so that we could begin to understand where we are, be prepared for not just another development, but a fully-fledged paradigm shift. We are moving beyond single-modality solutions that rely solely on text, images, or audio. The future lies in multimodal AI, a powerful approach that integrates data from various modalities to create a more comprehensive understanding of the world. This evolution necessitates a robust infrastructure capable of handling the complexities of processing and analyzing diverse data types.
In this article and ahead of the upcoming AI Infrastructure & Architecture Summit, we explore the rise of multimodal AI, why we need it, and how building sustainable multimodal systems can transform businesses by using diverse data types.
Multimodal AI: a Definition
Multimodal AI is a type of artificial intelligence that can process and understand multiple forms of data, such as text, images, audio and video. Unlike traditional AI systems that typically focus on a single data type, multimodal AI can integrate information from various sources to generate more comprehensive and accurate insights.
For example, in a hospital setting, a patient may present with symptoms that are difficult to diagnose. A multimodal AI system could be employed to analyze various forms of patient data simultaneously.
The system would process medical images (X-rays, MRIs, CT scans), patient records (electronic health records, lab results) and even audio data (heart sounds, breathing patterns). By correlating information from these different sources, the AI could identify patterns and anomalies that might be overlooked in a traditional, siloed approach.(X-rays, MRIs, CT scans), patient records (electronic health records, lab results) and even audio data (heart sounds, breathing patterns). By correlating information from these different sources, the AI could identify patterns and anomalies that might be overlooked in a traditional, siloed approach.
As Sarah Du explains in The Multimodal AI Revolution (Medium):
“We have open-sourced and closed-source models that handle text-to-speech (TTS/speech synthesis e.g. Play.ht, ElevenLabs, WellSaid Labs), speech-to-text (voice recognition e.g. Whisper), text-to-image (image generation e.g. Stable Diffusion, Midjourney, Imagen, DALL-E), image-to-text (image captioning e.g. GPT-4V, LLaVA), text-to-video (video synthesis e.g. Sora), and video-to-text (video transcription). As these models continue to improve, they become increasingly “good enough” to support and augment humans.”
The Rise of Multimodal AI
Traditional AI systems have largely focused on individual modalities. For example, image recognition analyzes visual data to identify objects in photos. This is fine as far as it goes, but the real world rarely presents information in such isolation. Humans naturally perceive the world by combining the various senses of sight, sound, touch and smell. Multimodal AI emulates this human capability, analyzing data such as text, images, audio and video simultaneously, leading to a more nuanced understanding of complex situations. The benefits of multimodal AI are numerous.
Here are a few key advantages:
- Enhanced Context and Accuracy: By analyzing data from multiple sources, multimodal models can glean deeper insights and improve decision-making. For example, in sentiment analysis, combining text with facial expressions can provide a more accurate understanding of a person's true emotions, allowing modification of response.
- Improved User Experiences: Multimodal interfaces allow users to interact with AI systems using a natural combination of speech, gestures and text, creating a more intuitive and engaging experience. Interaction between humans and computers have been proscribed by the human’s ability to code. We have progressed through natural language and are now able to add more subtlety to these conversations (as they have become).
- Revolutionizing Industries: From healthcare diagnosis based on medical images and patient records to autonomous vehicles that interpret their surroundings using sensors and cameras, multimodal AI holds the potential to transform various industries.
Why Do We Need Multimodal AI?
Much of the AI currently in use is based around Large Language Models (LLMs). As this article explains, there are issues with LLMs that stop organizations from fully harnessing the potential of AI tech:
- Accuracy and Reliability: Trained on hundreds of terabytes or even petabytes of unfiltered internet data, LLMs often generate incorrect or misleading information, known as hallucinations. This unreliability restricts their use in critical decision-making processes.
- Data Recency and Relevance: LLMs frequently struggle with providing up-to-date and relevant information. Their reliance on historical data limits their ability to address contemporary issues or access proprietary organizational data.
- Lack of Specialization: Generically trained LLMs often fall short in specific tasks due to their broad scope. Tailoring these models to excel in niche areas requires significant fine-tuning and additional data.
- Limited Adaptability and Control: Off-the-shelf LLMs offer minimal customization options, hindering their integration into diverse workflows. Users often resort to extensive prompt engineering to achieve desired outputs, which is inefficient and inconsistent.
The limitations of current LLMs underscore the need for a more sophisticated AI approach. To overcome the challenges highlighted above, the development of multimodal AI is a logical next step. Incorporating diverse data modalities gives models access to richer information, enhancing their ability to understand context, generate more accurate and relevant outputs and adapt to specific tasks. This evolution will be crucial for unlocking the full potential of AI and driving innovation across industries.
The Infrastructure Challenge
To realize the promise of multimodal AI, organizations must build a robust infrastructure founded on the following key pillars:
- High-performance Computing (HPC): Training and deploying multimodal models often require immense computational power. Scalable HPC resources, including powerful GPUs and specialized AI accelerators, are crucial for efficient data processing across different modalities.
- Data Storage and Management: Multimodal data encompasses a range of different formats, from large text documents and high-resolution images to lengthy video recordings. Building a scalable and secure storage solution with efficient data management tools is not optional.
- Advanced Networking Infrastructure: Seamless communication between different components of the AI infrastructure is critical. High-bandwidth, low-latency networks capable of handling diverse data types ensure smooth data flow and model training efficiency.
Building the Ecosystem: Beyond the Hardware
While hardware forms the foundation, a thriving multimodal AI ecosystem requires more.
Here are some key aspects to consider:
- Software Tools and Frameworks: A range of software tools are needed for tasks like data pre-processing, model development and integration. Multimodal-specific frameworks are emerging to address the unique challenges of working with diverse data types.
- Talent and Expertise: Building a skilled workforce with expertise in multimodal AI, data science and MLOps (Machine Learning Operations) is crucial. Universities and research institutions need to adapt their curriculum to prepare professionals for this evolving field.
- Standardization and Interoperability: As the field matures, developing standardized data formats and ensuring interoperability between different tools and platforms will be essential for fostering collaboration and innovation.
Building a Sustainable Multimodal Future
The benefits of multimodal AI are undeniable. There are challenges, which organizations must address if they are to pull the maximum value from this technology.
The three major challenges are:
- Data Privacy and Security: Multimodal data often contains sensitive information. Robust security protocols and adherence to data privacy regulations are essential for building trust in this technology.
- Energy Consumption: The immense computational power required by multimodal AI raises sustainability concerns. Focusing on energy-efficient hardware and exploring renewable energy sources are critical for a sustainable future.
- Ethical Considerations: The capabilities of multimodal AI need to be developed responsibly. Ethical considerations like bias reduction and transparency are essential for ensuring ethical and trustworthy AI applications.
Conclusion: A Multimodal Future Beckons
The ability to analyze and understand information from multiple sources is reshaping the AI landscape. By building a robust infrastructure and addressing the associated challenges, we can unlock the immense potential of multimodal AI. This future holds exciting possibilities for various industries, empowering them to gain deeper insights, create enhanced user experiences and drive innovation. It is by working on both the technological infrastructure and the ethical frameworks that we can truly pave the way for a responsible and impactful multimodal AI future.
Explore more live at #AIInfraSummit!
Join us on January 13th-15th, 2025 at Hilton London Syon Park at the #AIInfraSummit where AI engineering leaders and infrastructure experts will come together to redefine how enterprises design, deploy, and scale AI-driven applications. As an attendee, you'll gain firsthand insights from experts on delivering enterprise-scale generative AI ecosystems through a purpose-built, full-stack platform. Learn how to manage AI compute resources effectively, meet AI demands at any scale with infrastructure designed for custom workloads, and stay ahead of the curve by adapting to the evolution of foundational AI models. This is a summit which will enable your teams to stay abreast of enterprise AI deployments and operational excellence. Book your seat online now.