Building Infrastructure Foundation to Scale and Optimize AI Effort
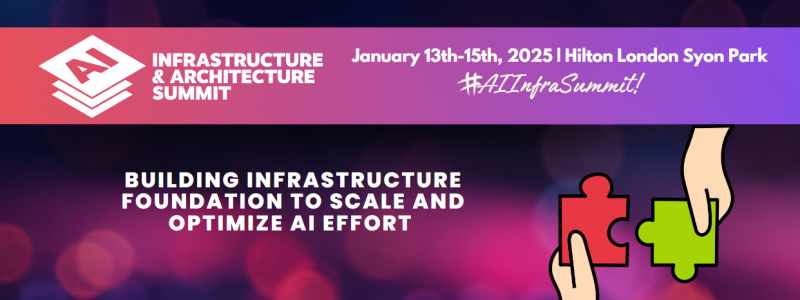
Artificial intelligence (AI) is rapidly transforming industries. From facial recognition unlocking smartphones to chatbots personalizing customer experiences, AI is already the main driver of emerging technologies such as big data, robotics and IoT.
According to IBM, enterprise AI adoption is on the rise, driven primarily by early adopters. Approximately 42 percent of large-scale companies have successfully implemented AI solutions, while another 40 percent are currently experimenting with the technology. A significant 59 percent of businesses which are exploring or deploying AI are accelerating their investments, indicating growing confidence in its potential. Despite this positive trend, obstacles such as a lack of AI expertise (33 percent), complex data management (25 percent) and ethical concerns (23 percent) continue to hinder broader adoption.
To truly realize the transformative power of AI, there must be the possibility of scaling and optimization. To achieve this, the challenge is for organizations to lay a strong foundation of robust infrastructure.
In his article and ahead of the upcoming AI Infrastructure & Architecture Summit, we explore will address these challenges and look at what businesses can do to move their successful early experiments in AI to a broader rollout across other departments.
The Need for a Scalable Foundation
Many organizations approach AI with a "proof-of-concept" mentality, developing small-scale models to demonstrate the technology's potential. Getting from zero to one, however, is not where the true value of AI lies; value is only realized when AI deployment grows from one to a hundred. AI must be able to be rolled out at scale and impact larger workflows. A simple example demonstrates why scaling is essential: imagine a fraud detection model that initially works well but buckles under the pressure of increased transaction volume. In every case with AI, without an infrastructure that can scale alongside your ambitions, the technology is of limited use and therefore of limited value.
There are three major pressures on technology that further point to why a scalable foundation is crucial for AI success:
- Growing Data Demands: AI models thrive on data and we are producing more data every day. As AI models become more complex and handle larger datasets, the underlying infrastructure needs to be able to efficiently store, process and analyze this data.
- Increased Model Complexity: Cutting-edge AI models often require immense computational power for training and deployment. A scalable infrastructure ensures you have the resources needed to train complex models that deliver superior results.
- Enhanced Agility and Adaptability: Machine learning, the core of AI, is an iterative process. Continuously refining and optimizing models is crucial for sustained performance. A scalable infrastructure allows for faster experimentation and deployment of improved models.
Building Blocks of a Scalable AI Infrastructure
Building a robust AI infrastructure requires careful consideration of several key components:
- High-Performance Computing (HPC): This refers to the hardware resources needed to train and deploy AI models. HPC typically includes powerful CPUs, GPUs (Graphics Processing Units) and potentially specialized AI accelerators. Choosing the right hardware configuration based on workload demands is essential for optimal performance and cost-effectiveness.
- Storage Infrastructure: AI models and data can be enormous. Building a scalable storage solution is essential. This might involve a combination of high-speed storage for real-time processing and cost-effective cloud storage for less frequently accessed data.
- Networking: Efficient and reliable communication between different components of the AI infrastructure is crucial. High-bandwidth, low-latency networks are needed to ensure smooth data flow and model training efficiency.
- Software Tools and Frameworks: A range of software tools are crucial for managing the AI lifecycle. These include libraries like TensorFlow and PyTorch for model development, containerization tools like Docker for efficient deployment and MLOps (Machine Learning Operations) platforms for automating the process of deploying and managing AI models in production environments.
Optimizing Your AI Infrastructure
Building a scalable foundation is just the first step. To truly optimize your AI efforts, additional considerations are needed:
- Resource Efficiency: While powerful hardware is essential, efficient resource utilization is going to make the difference to how well it works. Techniques such as workload scheduling and containerization can help optimize hardware utilization and reduce costs.
- Cloud Adoption: Cloud platforms offer a pay-as-you-go model for accessing High Performance Computing resources. This flexible approach allows organizations to scale their infrastructure based on specific needs, reducing upfront costs and offering additional benefits like global reach and disaster recovery capabilities. Digital Realty, for example, provides cloud-neutral data centers that can house your AI infrastructure, offering the flexibility to choose the cloud provider that best suits your needs.
- Collaboration and Best Practices: one of the most important things you can do in your efforts to optimize your infrastructure is a human rather than technological practice. Building a robust AI infrastructure is complex, but it has been done before and there is no need to reinvent the wheel: lean on and learn from others who have won battle scars on their own journeys. Insights from industry leaders, learning best practices and fostering collaboration within the AI ecosystem can provide valuable knowledge and accelerate progress.
Optimizing your AI infrastructure is essential for maximizing its potential and ensuring sustainable operations. By carefully balancing hardware resources, leveraging cloud-based solutions and fostering collaboration within the AI ecosystem, organizations can significantly enhance efficiency and reduce costs. Continuously evaluating and adapting your infrastructure to emerging technologies and best practices is crucial for staying ahead in the rapidly evolving AI landscape.
Continuous Improvement and Future Considerations
AI is a rapidly evolving field.As the technology matures, new solutions will emerge and existing infrastructure needs to be continuously evaluated and optimized.
Here are some additional factors to consider for future development:
- Security and Privacy: Securing sensitive data and ensuring the responsible development and deployment of AI models are critical issues, with the public becoming increasingly aware of - and alarmed by - the potential for data breaches that can have a global impact. Events such as the CrowdStrike IT outage demonstrated the potential for disruption. Putting that power into the hands of bad actors is a serious concern. Robust data security protocols and ethical guidelines need to be integrated into the AI infrastructure.
- Green AI: Wells Fargo projects the power demand of AI to surge from 8 TWh in 2024 to 52 TWh in 2026. They predict it will rise to 652 TWh by 2030 - a 8,050 percent growth from their 2024 projected level. The high energy consumption of AI infrastructure raises sustainability concerns. Focusing on energy-efficient hardware and harnessing renewable energy sources are crucial in creating a more sustainable AI future.
- Quantum Computing: The potential of quantum computing to revolutionize AI is immense. While still in its nascent stages, the technology holds the promise to dramatically accelerate AI development and capabilities. Quantum computers leverage quantum mechanics to perform calculations exponentially faster than classical computers, potentially enabling breakthroughs that could redefine the boundaries of what is computationally feasible. Currently intractable tasks, such as optimizing complex systems or developing highly accurate AI models, could become routine. Additionally, quantum computing has the potential to address challenges in machine learning, such as improving the efficiency of training deep neural networks and developing more robust algorithms.
The future of AI infrastructure is inextricably linked to a complex interplay of technological advancements, ethical considerations and sustainability. As AI continues to evolve, organizations must remain agile, adopting new solutions and optimizing existing infrastructure to meet the demands of increasingly complex models. By prioritizing data security,energy efficiency and responsible AI development, businesses can harness the full potential of AI while mitigating its risks. Ultimately, the successful integration of these factors will be crucial for building a sustainable and ethical AI future.
Conclusion: A Strong Foundation for AI Success
Building a scalable and optimized AI infrastructure is foundational to unlocking the true potential of AI. By carefully selecting hardware components, adopting cloud-based solutions and continuously optimizing resource utilization, organizations can empower their AI initiatives, leading to enhanced performance, cost-efficiency and long-term success. As we move forward, the focus should be on not just optimizing the infrastructure but also ensuring ethical, responsible and sustainable development of AI. By addressing these challenges and embracing emerging technologies like quantum computing, we can create a future where AI benefits society as a whole.
Ultimately, a robust AI infrastructure is not just about technology; it's about enabling innovation, driving business growth and creating positive impact.
Explore more live at #AIInfraSummit!
Join us on January 13th-15th, 2025 at Hilton London Syon Park at the #AIInfraSummit where AI engineering leaders and infrastructure experts will come together to redefine how enterprises design, deploy, and scale AI-driven applications. As an attendee, you'll gain firsthand insights from experts on delivering enterprise-scale generative AI ecosystems through a purpose-built, full-stack platform. Learn how to manage AI compute resources effectively, meet AI demands at any scale with infrastructure designed for custom workloads, and stay ahead of the curve by adapting to the evolution of foundational AI models. This is a summit which will enable your teams to stay abreast of enterprise AI deployments and operational excellence. Book your seat online now.