How Businesses are Finding Ways to Bring Down the High Cost in AI Infrastructure
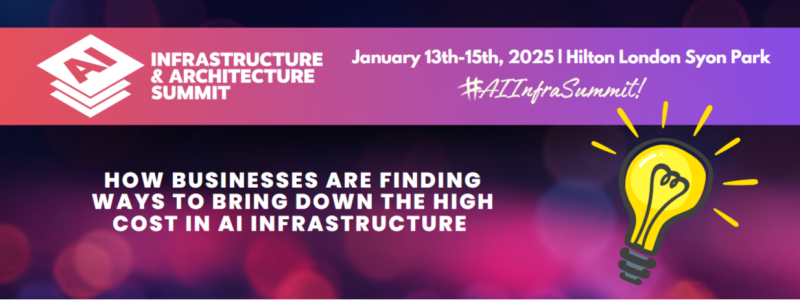
The transformative power of artificial intelligence (AI) has many business leaders excited with anticipation. Exploring the potential of this technology has become a high priority for every industry hungry to apply it in practical ways: from revolutionizing healthcare with targeted drug discovery to optimizing logistics with predictive maintenance, AI offers a treasure trove of possibilities across every sector.
The onward march of AI seems unstoppable, but there is one major hurdle that continues to impede widespread adoption: the high cost of AI infrastructure. The computational power required to train and run complex AI models requires significant investments in hardware, software and expertise. This article delves into practical ways to bring down the high cost of AI infrastructure, paving the way for a more accessible and impactful AI future.
In this article and ahead of the upcoming AI Infrastructure & Architecture Summit, we explore the power of AI and how businesses are innovating to reduce the high costs related with AI Infrastructure.
Understanding the Four Cost Factors: A Breakdown
One source states that there are instances of companies spending in excess of 80 percent of their total capital raised on compute resources to feed their AI efforts - a huge and perhaps not sustainable allocation of resources. Before exploring cost-reduction strategies, then, it's crucial to understand what drives the high cost of AI infrastructure. There are four key cost factors that make up the bulk of AI infrastructure spending:
- Hardware: Training and deploying AI models often requires high-performance computing (HPC) resources, including powerful CPUs, GPUs (Graphics Processing Units) and specialized AI accelerators. These components can be expensive to acquire and maintain, especially for organizations with complex AI needs.
- Software: AI development necessitates a range of software licenses, from cloud-based platforms such as TensorFlow or PyTorch to specialized tools for model optimization and deployment. These licenses can accumulate over time, adding significantly to the overall cost.
- Expertise: Building, training and managing AI models requires a specialized skillset, encompassing data science, machine learning engineering and MLOps (Machine Learning Operations) expertise. Hiring and retaining these professionals can be expensive, particularly for smaller organizations.
- Energy Consumption: The immense computational power used in AI translates into high energy consumption. This not only impacts operational costs but also raises concerns about environmental sustainability.
The title “AI infrastructure” misleadingly suggests something that can be built and then left to run; you will notice that many of the above four cost factors are ongoing. Even hardware may need to be regularly replaced to keep pace with developing trends. Therefore it is essential that AI infrastructure delivers an ROI to justify the effort of building it in the first place.
Strategies for Cost Optimization: Taming the AI Infrastructure Beast
Fortunately, various strategies can help organizations bring down the cost of AI infrastructure, making this transformative technology more accessible:
- Right-sizing Hardware: Many organizations may be tempted to overprovision hardware, leading to inefficient resource utilization. Careful analysis of AI workloads and choosing the right hardware configuration for specific tasks can significantly reduce costs. The Carnegie Mellon University Software Engineering Institute (SEI) emphasizes the importance of "right-sizing" hardware, suggesting cloud-based pay-as-you-go models and exploring resource optimization tools.
- Leveraging Cloud-based AI Platforms: Cloud platforms such as Google Cloud AI Platform, Amazon SageMaker and Microsoft Azure Machine Learning offer pre-configured environments for training and deploying AI models. These platforms provide access to scalable resources and pay-as-you-go billing models, reducing upfront costs and offering flexibility in scaling resources based on needs.
- Optimizing Model Efficiency: Not all AI models are suitable for every organization. Large, complex models can be particularly resource-intensive. Focusing on model architecture optimization techniques such as pruning and quantization can significantly reduce the computational requirements of a model without compromising its accuracy. Techniques such as knowledge distillation, where a smaller model learns from a larger, pre-trained one, can also help achieve better efficiency.
- Adopting MLOps Practices: MLOps streamlines the machine learning development lifecycle, focusing on automating repetitive tasks and improving data and model management. By automating model deployment and monitoring, MLOps can help identify and eliminate resource inefficiencies. As highlighted in a Medium article by Nick Hystax, MLOps can lead to cost savings by ensuring resource optimization and proactive model performance monitoring:
“The efficient management of ML processes is crucial for reducing infrastructure costs. By optimizing resource allocation, scheduling jobs during off-peak hours, containerizing processes, and monitoring and optimizing performance, companies can reduce the overall infrastructure costs associated with ML without sacrificing performance or functionality.”
- Open Source Tools and Frameworks: The open-source community has been instrumental in democratizing AI, providing a wealth of tools and resources that accelerate development and reduce costs. By leveraging open-source frameworks like TensorFlow, PyTorch and scikit-learn, organizations can significantly curtail software licensing expenses. These platforms offer a comprehensive suite of tools for tasks ranging from data preprocessing and visualization to model building, training, and deployment. Beyond cost savings, open-source tools promote transparency, collaboration, and rapid advancement.
The open-source nature of these platforms encourages knowledge sharing, allowing developers to learn from each other and build upon existing work. This collaborative environment accelerates the pace of AI innovation and ensures that the benefits of AI are widely accessible.
- Collaborative AI Development: By fostering collaborative relationships, research institutions and businesses can pool resources, expertise, and data to drive groundbreaking innovations. This synergistic approach enables the sharing of best practices, the development of common standards, and the acceleration of research-to-market timelines.
Joint research projects, where academia provides theoretical underpinnings and industry contributes real-world data and computational resources, can yield significant breakthroughs. Additionally, the establishment of shared infrastructure resources, such as high-performance computing facilities or data platforms, can streamline collaboration and reduce costs for both parties.
Beyond Cost Reduction: Sustainability Considerations
While cost reduction is a critical objective, another crucial aspect to consider is the environmental impact of AI infrastructure. High energy consumption associated with AI training and deployment raises sustainability concerns.
There are promising technological advancements helping to address this issue. Specialized hardware architectures, such as AI accelerators designed for energy efficiency, are becoming more prevalent. Additionally, utilizing renewable energy sources for powering AI infrastructure allows for more environmentally responsible development and deployment.
Conclusion: A Sustainable and Cost-Effective Future for AI
The high cost of AI infrastructure presents a significant hurdle. With the strategies outlined above, however, organizations can pave the way for a more accessible and sustainable AI future. By optimizing hardware resources, leveraging cloud platforms and adopting MLOps practices, costs can be brought down, allowing more organizations to unlock the transformative potential of AI. Furthermore, focusing on green AI practices such as energy-efficient hardware and renewable energy sources ensures a sustainable future for this powerful technology. By addressing both cost and environmental concerns, we can unleash the full potential of AI while minimizing its negative impacts.
As AI continues to evolve, it is essential to remain adaptable and explore emerging technologies that can further reduce costs and improve efficiency. For instance, advancements in quantum computing hold the potential to revolutionize AI by offering unprecedented computational power. Additionally, ongoing research in AI algorithms and model optimization will likely lead to more efficient and cost-effective solutions.
Finally, by fostering collaboration between academia, industry and government, we can accelerate the development of AI technologies while ensuring they are accessible and beneficial to society as a whole. Through a concerted effort to address the challenges of cost and sustainability, we can unlock the full potential of AI to drive innovation, economic growth and positive social impact.
Explore more live at #AIInfraSummit!
Join us on January 13th-15th, 2025 at Hilton London Syon Park at the #AIInfraSummit where AI engineering leaders and infrastructure experts will come together to redefine how enterprises design, deploy, and scale AI-driven applications. As an attendee, you'll gain firsthand insights from experts on delivering enterprise-scale generative AI ecosystems through a purpose-built, full-stack platform. Learn how to manage AI compute resources effectively, meet AI demands at any scale with infrastructure designed for custom workloads, and stay ahead of the curve by adapting to the evolution of foundational AI models. This is a summit which will enable your teams to stay abreast of enterprise AI deployments and operational excellence. Book your seat online now.