Carnegie Mellon University Use AI To Try and Solve Humanity’s Battery Problem
The research uses a combined robotic and artificial intelligence system to design better electrolytes for lithium-ion batteries.
Add bookmark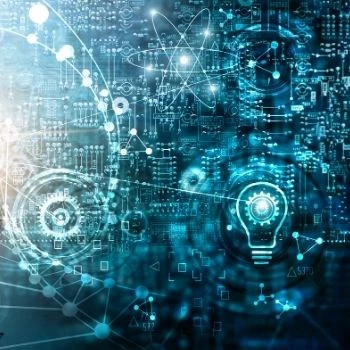
The world has a battery problem. As we continue to increase our reliance on technology, we require the resources and materials to create more batteries than ever before. On top of that, despite increasing reliance on batteries over recent decades, battery technology has failed to keep up the pace of advancement in other areas – stumbling behind other advancements in terms of efficiency and charging capability.
However, according to a paper recently published in Nature Communications, researchers from Carnegie Mellon have used a combined robotic and artificial intelligence system to design better electrolytes for lithium-ion batteries.
In particular, the team was looking for electrolytes that would allow for batteries to charge faster—one of the biggest problems in battery technology today and a major barrier to widespread electric vehicle adoption.
Lithium-ion batteries have a cathode and an anode surrounded by an electrolyte. When they are charged, ions migrate through the electrolyte from the cathode to the anode (and vice-versa when they discharge). How fast a battery charges and discharges depend on the exact composition of the electrolyte. Optimizing the electrolyte solution is one of the key challenges for battery designers, and until now research had relied on guesswork, intuition, and trial and error.
As the paper points out, “There are many choices for solvent17,18,19 or salt20,21, each potentially yielding vastly different performance; optimized electrolyte solutions often contain more than three or four components”. This is why “battery innovations can take years to deliver”, because there are so many potential chemicals that can be used in various ratios that optimizing them is “time-consuming and laborious”, until now.
According to the research paper, the team used an arrangement of automated pumps, valves, vessels and other lab equipment (which they named ‘Clio’) to mix together different ratios of three potential solvents and one salt at a significantly faster rate to traditional methods of research.
Clio’s results were fed into a machine-learning system dubbed “Dragonfly” that analyzed the data to look for patterns and propose alternative ratios that might perform better. Clio then automatically ran those new proposed experiments, allowing for Dragonfly to optimize the chemical recipes yet further.
In total, working with just the one salt and three solvents, Clio and Dragonfly were able to run 42 experiments over two days and come up with six solutions that out-performed an existing electrolyte solution made from the same four chemicals. The best test cell containing one of the robot-AI-developed electrolytes boasted a 13 percent improvement in performance compared to the best performing test cell using the commercially available electrolyte.
In this case, Clio and Dragonfly were optimizing for recharge speed, but similar “closed-loop” experiments could optimize for capacity, discharge time, voltage, and all the other factors that matter in commercial battery performance.
And the potential expands far beyond the world of batteries. In fact, the team thinks their work will “be useful beyond the battery community,” claiming that their “custom-designed robotic platform, experiment planning, and integration with device testing will be valuable in optimizing other autonomous discovery platforms for energy applications and material science in general.”
This new research just further shows the transformative power of AI and associated technologies, by adopting machine learning techniques, enterprises across all industries are discovering the supercharging power of AI, data and analytics.